The digital marketing landscape is in a state of constant, rapid evolution, demanding more than just raw data—it requires actionable intelligence. As attaining these actionable insights has become increasingly difficult, Google Analytics (GA) has continued to evolve through building artificial intelligence (AI) into the platform’s foundation. With AI at the core of GA, organizations are more easily able to automate complex tasks, enhancing attribution capabilities, revealing hidden patterns, and providing predictive intelligence. This infusion of AI transforms GA from a mere reporting tool to a strategic platform organizations can leverage in day-to-day workflows.
Through our “Mastering AI For Marketing” article series, we have explored how to optimize content for AI visibility, what the future of paid marketing via AI might look like, and methods to deploy for analyzing AI-driven traffic. In this article, we will explore the key AI features available in GA that are revolutionizing how marketers and analysts approach their daily tasks, ultimately enhancing efficiency and driving better decision-making.
Data Processing
As mentioned in the intro, GA leverages AI and machine learning not only for reporting and activation purposes, but also at the very foundation of the tool when the data is being processed. While the data is being processed, GA deploys data modeling in a number of ways to enhance the data that is collected during the processing period to help reduce data gaps, enhance attribution, and provide the ability to glean actionable insights. Currently, in GA, there are four different ways AI may be deployed during the data processing period.
- Key Event Modeling – Deployed to help estimate online key events that can’t be directly tied back to a specific channel for attribution purposes. This modeling enables organizations to more accurately attribute key event completions when the user is not able to be identified due to user privacy, technical limitations (cookie restrictions, Apple ATT and ITP, and browser extensions), or users moving between devices. This modeling capability is on by default and will primarily re-attribute traffic from direct channels to other channels based on trends that can be observed and attributed correctly. It is important to note that this kind of data modeling may take up to 12 days to fully complete from when the conversion is recorded.
- Behavioral Modeling – Estimates behavioral metrics when no identifiable analytics cookies can be set or accessed from the user’s browser. This may be due to the user denying consent or due to the default requirement put in place by local privacy regulations. This modeling capability is activated when advanced consent mode is activated for anonymous event tracking purposes. Advanced consent mode is typically activated to help reduce data gaps caused by the collection of anonymous event tracking. Activating advanced consent mode is typically recommended as a last resort when it comes to adhering to privacy laws, as there are a few thresholds that need to be met before modeled data can be made available in reports. To activate behavior modeling via advanced consent mode, users need:
- Events: 1000 unconsented events/interactions per day for the last 7 days
- Daily Users: 1000 consented users for at least 7 of the previous 28 days
- Forecast Modeling – Also known as predictive metrics, models predict future user behavior relating to probability of the user’s likelihood of falling into the category or completing an action. These metrics can be applied to reports and also when building audiences. This feature is not as heavily utilized, but when deployed correctly can unlock potential, especially for mid-small organizations. More features related to forecasting are expected to come out in the future as there is large potential for how this technology can be leveraged and deployed. There are also requirements necessary to meet the necessary threshold to leverage this modeling capability.
- Attribution Modeling – Better known as Data-Driven Attribution, this model attempts to more comprehensively attribute key event completions across channels that impact the user’s journey across devices and platforms. This helps ensure more holistic reporting regarding attribution, even when observable data is not available due to cookie loss or measurement restrictions. When leveraging this modeling capability in GA, the model assigns key event completion credit to marketing touchpoints seen across the user’s journey. This is completed by identifying how the addition of different touchpoints to a user’s journey changes the probability of a key event(s) being completed. This model is activated when the Reporting Attribution Model is updated by accessing the Attribution Setting of the GA property. It is also important to note that this forecasting only affects attribution at the event scope level, as seen below with an example of a user’s journey.
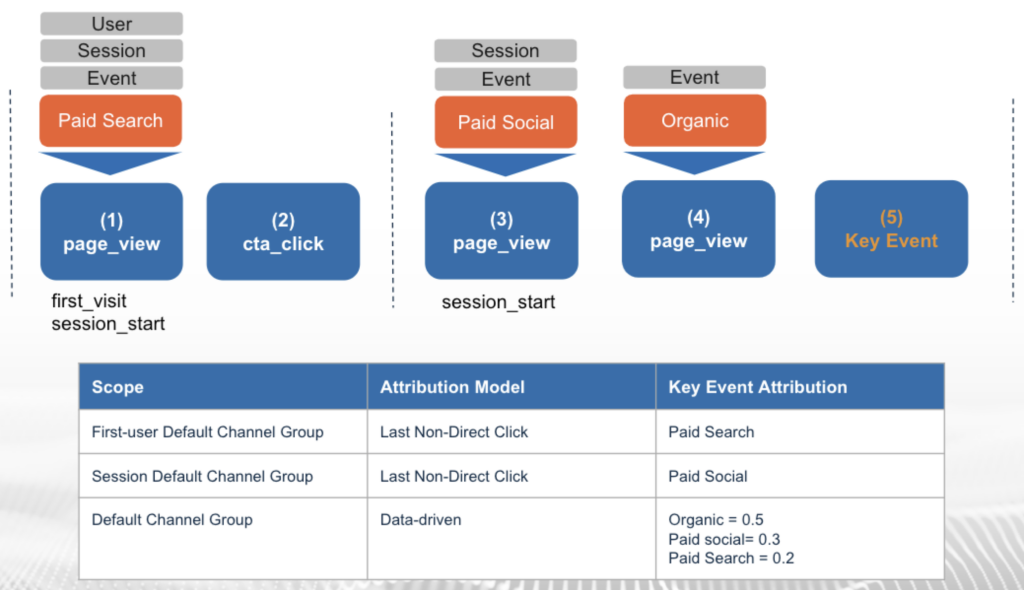
Before activating some of these models, it is important for organizations to determine which side of the scale they fall on in relation to data enhancement and data definition. While we all wish any feature we activated would only improve our data, it is important to keep in mind that there are tradeoffs that occur. The more models that are activated, the more your organizations data is sent through black boxes that you don’t control. Thus, if someone were to ask how the exact values reported were attained, there wouldn’t be an option to open up the model and explain the details. Instead, organizations have to rely on what is said to be happening via GA documentation and trust something they may not have much insight into. However, without activating these models, the explanation of why data is reported the way it is becomes much easier, but it also means that the data being accessed is very basic.
Recommendation
The recommended approach for organizations to take is to leverage attribution modeling, as this will help organizations better understand the impact of their marketing efforts. However, validating that this is working soundly will be vital. Organizations can accomplish validation of the model by comparing attribution models in the advertising reports section. If data seems wildly off or doesn’t make sense, then it is best to investigate and potentially move away from leveraging this modeling feature for a time.
Leveraging behavior modeling is a little more of a case-by-case basis and should be treated as such. The reason for this is it ultimately depends on the region(s) the organization operates in, the legal counsels interpretation of privacy regulation, and the anticipated unconsented rate of users. All of the above varies substantially across organizations, and more importantly, can be affected by external guidelines and factors. Additionally, organizations can quickly exclude behavioral modeling once it has been activated by simply changing the GA reporting identity of the property. This ensures that organizations who do active behavioral modeling are able to validate the data collected via Advanced Consent Mode retroactively.
Lastly, as mentioned above, forecast modeling is something that may be relatively new to many organizations, but can be very useful to small-mid sized organizations. It is recommended that organizations test out the predictive capabilities that GA offers by building a small test campaign and observing how it performs compared to other remarketing audiences. For larger organizations, building out their own predictive capabilities like propensity modeling or customer lifetime value analysis are likely more feasible and offer more control to the organization.
Data Reporting
In addition to data processing, GA also enhances data reporting capabilities within the UI experience. The built-in AI helps organizations navigate, identify, and report important data insights. As data is ingested into GA, these AI-backed features continue to become more enhanced and provide more accurate recommendations as the models are trained. A majority of the features that are available are also easy to leverage and do have some customization capabilities.
- Analytics Insights – One AI data reporting capability is the ability to generate unique reporting on oddities or outliers. This is completed through the use of three different capabilities (anomaly detection, trend analysis, and contribution analysis) ultimately being rolled up into one feature known as Analytics Insights. Organizations can either let GA identify these insights for your organization or they can also set up their own customized insights that can be utilized for providing alerts. These insights will populate through cards on the home page and snapshot reports under the Insights session or by selecting the insights icon in the right-hand corner of the standard reports.
- Anomaly Detection – Leverages a Bayesian-state-space time series model on historically collected data to help predict data anomaly that occur during a given period. This detection is run on metrics that are available in GA. These anomalies that are identified by monitoring abrupt spikes or changes in the data. It is also important to note that this anomaly detection is also deployed on other time-series graphs seen through the GA reporting interface, such as explore reports.
- Trend Analysis – Similar to Anomaly Detection, trend analysis is instead built to look for longer lasting persistent trends that occur across metrics. The primary goal of trend analysis is to identify when subtler, longer-lasting changes occur that are out of the normal compared to historical data trends. GA deploys an algorithm called signal segmentation to detect these changes in the trend.
- Contribution Analysis – Provides details, via statistical techniques, to identify user segments that are contributing to the anomalies. These user segments are best thought of as characteristics of the user, such as location, attribution, age range, specific event completions, etc.
- Recommendations – Organizations may also see additional messages populate in the Insights section that encourage further adoption of various features that may not be fully utilized in the platform. These messages are called recommendations and will show up periodically for personalized feature adoption recommendations.
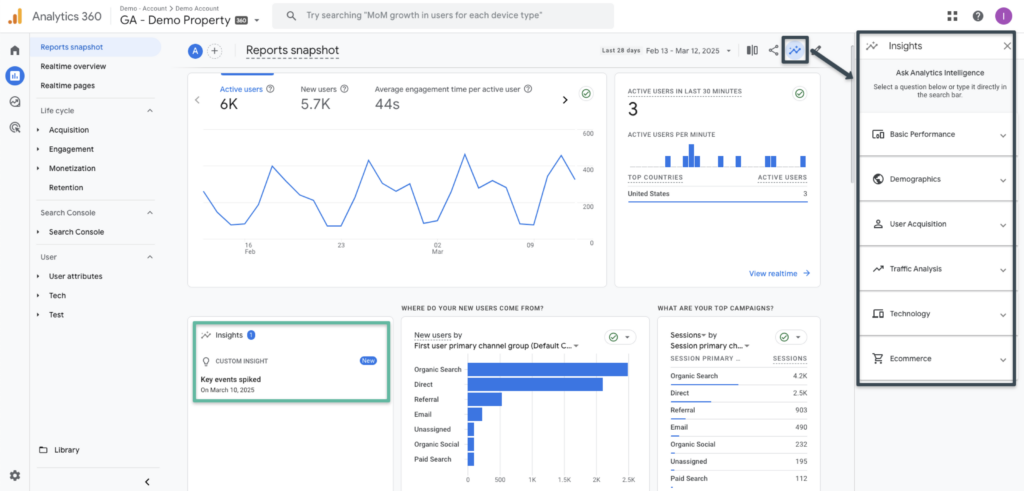
- Generated Insights – Similar to the above, generated insights enables insights to be raised concerning data from the specific report that the organizations are viewing. These insights are generally more detailed than the analytics insights seen above, but are easily digestible. Thus, the generated insights can be helpful by providing organizations with unique insights that might otherwise not be easily identified, including what data points changed the most in the last month and what GA configuration updates/enhancements may have contributed to the change in the data points.
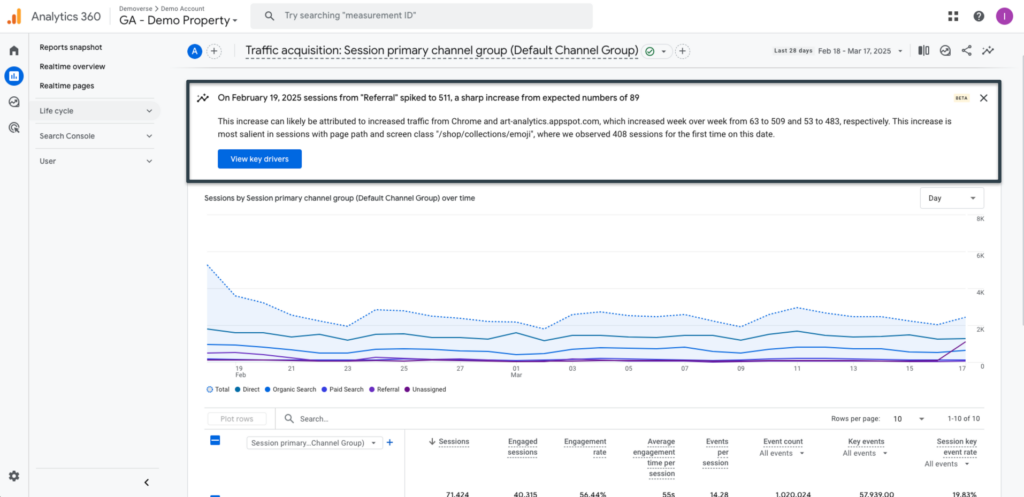
- Natural Language Search – Enables organizations to leverage the search feature via natural language, but receive tailored details and information related to the inquiry. This can be extremely beneficial if there is a detailed question about the data, but the organization is unsure where to start or what report may help answer the questions.
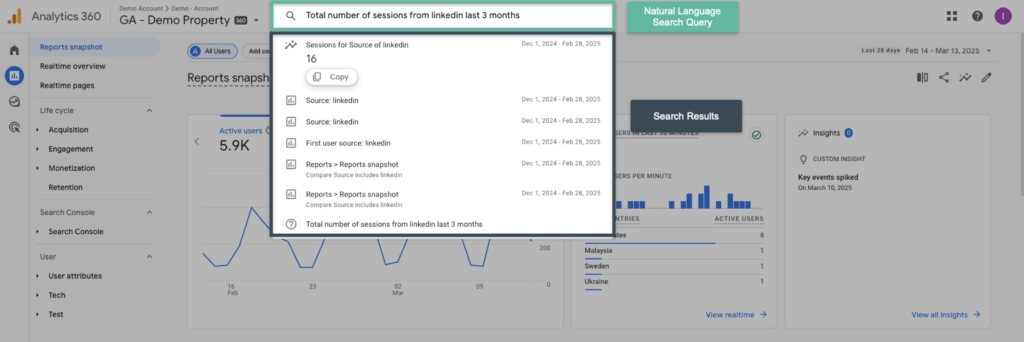
- Benchmarking – While it may not be thought of as an AI feature in GA, benchmarking is a feature that comes as a result of GA’s use of AI to determine percentiles of the data related to the industry. Benchmarking provides organizations with the ability to compare important business metrics to other organizations’ aggregated data in their industry/peer group. This enables organizations to better identify potential optimization opportunities related to their platform that may help contribute to improving performance.
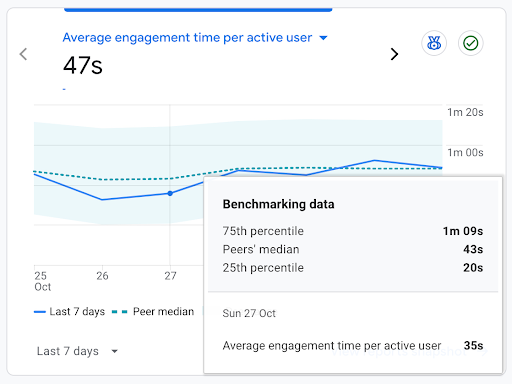
Recommendation
Enhancing reports through AI will only continue to improve as more AI features are released to GA. Ensuring your organization knows how to take advantage of the features that are currently available will only help the adoption of new AI features that are surely to be released to the product in the future. Setting up customized analytics insights to alert the organization about anomalies or trends that impact business KPIs and what contributed to the anomalies will help organizations be more proactive about acting based on what the data collected is saying. However, don’t just stop there; once these insights are triggered, continue to dive deeper into the insights identified and try to understand the correlation that may exist and why the insight was triggered.
Data Activation
The final area we will explore is data activation and how AI is being utilized to enhance the data activation capabilities of organizations. While this is also an area that expects a lot of enhancements as well, there are currently a few features to note that can be leveraged to improve an organization’s data activation today.
- Predictive Audiences – One feature that is native to GA is predictive audiences. This feature enables organizations to create an audience leveraging one of the predictive metrics features we discussed earlier in this article. This feature is once again recommended for small-mid-sized organizations that may have a little budget and time to test out how these audiences perform compared to traditional audiences built. Audiences categories that can be built include likely purchasers, churning purchasers, and predicted top spenders. Organizations can specify the probability range related to the predicted metric or use the pre-built ranges.
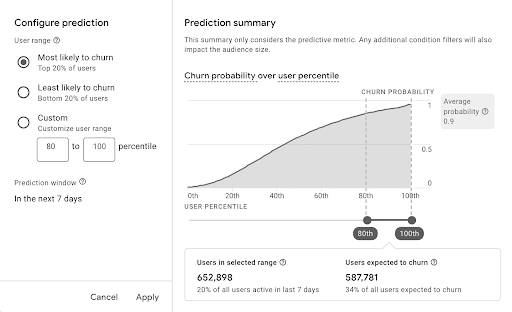
- Meridian – Leveraging additional tools and models developed by Google to help improve data activation based on GA data is also a great alternative to the UI. Meridian is a recently released marketing mix model (MMM) that helps organizations measure campaign performance to inform budget planning decisions and improve media effectiveness. It completes this by leveraging a highly customizable modeling framework based on Bayesian causal inference. While the data is aggregated to ensure the model is privacy-safe, this MMM model can still account for both non-marketing and channel factors that impact business KPIs.
- BigQuery – One of the most effective data activation routes and organization can take is leveraging AI to export GA data to BigQuery. Once the data is in BigQuery, users can leverage features like Vertex AI or BQML to create their own models that are more personalized to their business and outcomes. While GA offers many AI features, there are limitations that exist due to the platform being third-party and restricted based on privacy regulations. Since BigQuery doesn’t have this limitation, there is more freedom to leverage CRM and other unique user data to create robust models.
Recommendation
Exploring what data activation looks like for your organization and how AI can be deployed to further assist with this process is vital. Out of the three sections discussed here, data activation is one of the core areas where organizations may see a significant uplift in capabilities and revenue generation. Organizations that have the ability to build their own models and leverage their backend data will see a competitive advantage over other organizations through increased ROAS and ROI, but setting aside funding to explore these options will need to come first.
Top 4 Recommendations for Leveraging Google Analytics’ Built-In AI Features
- Implement and Validate Data-Driven Attribution Modeling:
- Action:
- Activate data-driven attribution in your GA property’s Attribution Settings.
- Regularly compare attribution models in the advertising reports section to validate the accuracy and effectiveness of the data-driven model.
- If discrepancies arise, investigate and potentially adjust or temporarily disable the model.
- Why: This action directly addresses the most recommended modeling feature, allowing for a more comprehensive understanding of marketing efforts across channels and devices.
2. Set Up Custom Analytics Insights for Key Performance Indicators (KPIs):
- Action:
- Create custom Analytics Insights to monitor critical KPIs, focusing on anomaly detection, trend analysis, and contribution analysis.
- Configure alerts to be notified of significant deviations or trends.
- Proactively investigate and respond to insights, taking action based on identified anomalies or trends.
- Why: This empowers organizations to move from reactive to proactive data analysis, enabling timely responses to changes in performance and optimization opportunities.
3. Explore and Test Predictive Audiences for Targeted Marketing Campaigns:
- Action:
- Utilize GA’s Predictive Audiences feature to create targeted audiences based on predictive metrics like purchase probability and churn probability.
- Run A/B tests comparing the performance of predictive audiences against traditional audiences in marketing campaigns.
- Adjust audience parameters and campaign strategies based on performance results.
- Why: This allows smaller to medium-sized companies to test the value of predictive metrics, and create more effective marketing campaigns that are tailored to the user.
4. Evaluate and Plan for Advanced Data Activation with BigQuery:
- Action:
- Assess the organization’s capabilities and resources for leveraging BigQuery for advanced data activation.
- If feasible, explore exporting GA data to BigQuery and utilizing tools like Vertex AI or BQML for custom model development.
- Develop a strategy for integrating CRM and other backend data to enhance model accuracy and personalization.
- Why: This action addresses the highest level of data activation, offering significant potential for competitive advantage through personalized models and enhanced ROI.
Conclusion
The digital landscape is shifting, and GA, powered by AI, is your key to staying ahead. By strategically implementing AI-driven modeling, you can uncover hidden patterns and gain a deeper understanding of your customer journeys, giving you a distinct competitive advantage. Imagine knowing which touchpoints truly drive conversions, or predicting customer behavior to personalize your marketing efforts.
While AI offers immense potential, it’s crucial to approach it with a strategic mindset. Validate your modeled data, set up custom insights to monitor your KPIs proactively, and test predictive audiences to refine your campaigns. Don’t just react to data; anticipate trends and seize opportunities. For those ready to innovate, BigQuery offers the chance to build truly bespoke AI-powered marketing strategies.
This isn’t about simply keeping up—it’s about leading the way. By embracing the AI capabilities within GA, you can transform your data into a powerful asset, driving growth and maximizing your marketing ROI. The tools are here; the opportunity is now. Let’s make your data work smarter, not harder.