As Google Analytics 4 (GA4) users continue to build out reporting and analytics assets, leveraging the correct product is key to ensuring success. There are many different visualization and data storage options available on the market today with some organizations choosing to use a mixture of both. You might be thinking, “What platforms are most heavily utilized by other organizations on GA4?” or “What combination of products tend to provide the best results with GA4 data?”
Now while these questions are important and definitely would be helpful (maybe with enough interest we can dive further into these questions), I am instead going to suggest taking one step back to how users should extract data from GA4. The reason for this is because it will determine the limits and capabilities that will be faced.
Google Analytics Data Extraction
When extracting data from GA4, there are currently three ways to do so: download as a file, connect to the Google Analytics Data API (GA4), or connect to BigQuery (BQ). All three of the solutions are viable depending on the project’s size and scope. In today’s article, we will be focusing on some of the use cases and limitations of the three options.
File Download
The most limited of the three options above, downloading a file from the GA4 UI is typically utilized if a user is trying to complete some simple data extract from a standard report (CSV or PDF) or explore reports (Google Sheet TSV, CSV, or PDF). Typically, the scope of these projects are very small and are a result of an interest in doing some quick data analysis or not wanting to be limited by the max rows being shown in the GA4 UI.
- Pros:
- Easy and straightforward to use.
- Fastest way to get access to GA4 data (when data sets are small).
- No cost associated with downloading reports.
- Can be accessed in the GA4 UI.
- Cons:
- Data limited to what is available in the GA4 UI report.
- Downloading multiple reports will require multiple downloads and files.
- Large data sets require a long period of time to download and may fail.
- Downloaded data is static and does not update.
- Restricted to the date range selected in the report.
- Sampling, cardinality, and thresholding may affect the data (GA 360 licensed accounts do have access to unsampled reports and expanded data set capabilities).


Google Analytics Data API (GA4)
Google Analytics Data API (GA4) is likely the most heavily utilized data extraction capability of the three options. It is used by all third-party data transfer tools (Supermetrics, FiveTran, OWOX, etc.) and any visualization tools (Looker Studio, Tableau, Power BI, etc.) that integrate/connect directly with GA4. Connecting to the Google Analytics Data API (GA4) is typically best for organizations who want to report on aggregate data that is available and seen in the GA4 UI. This might include medium-sized projects or projects that don’t include a need to access more unique data analytics capabilities.
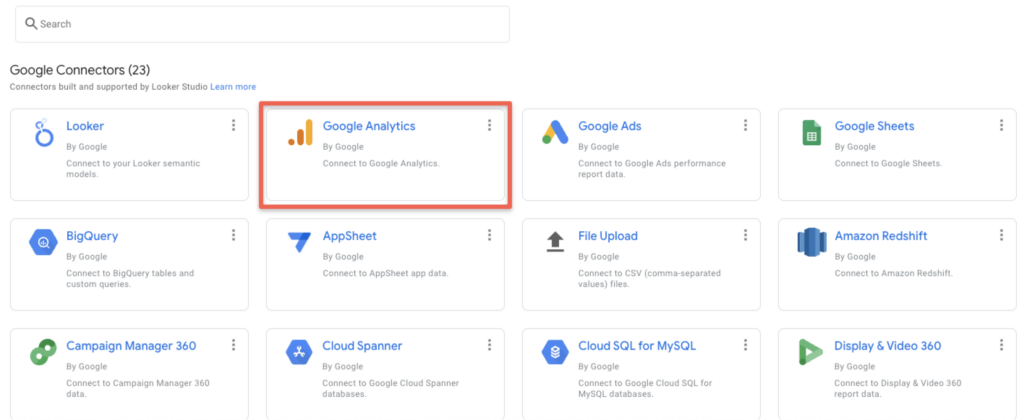
- Pros:
- Does not require SQL or coding experience to leverage.
- Easiest way to connect and extract large amounts of data from the GA4 UI.
- Is tool-agnostic and is able to be used by a variety of tools and programming languages.
- Free to users who wish to use the API Connector (limits do apply, other products may charge to connect).
- Can be accessed by developers and data scientists through API calls.
- Not limited to what is available within a specific report (can combine data similar to the explore reports.
- Historical data available immediately once connected (date range depends on data retention setting).
- Cons:
- Data is restricted to what is available in GA4 (if a parameter is not registered in the GA4 UI, then data won’t be pulled through).
- Key IDs and data such as timestamp and user_id are unavailable to access.
- Date range for pulling data is restricted to the data retention settings of the GA4 property.
- No access to the raw unsampled/queried data.
- Is subject to analytics property quotas as seen below:
Quota Name | Standard Property Limit | Analytics 360 Property Limit |
---|---|---|
Core Tokens Per Property Per Day | 200,000 | 2,000,000 |
Core Tokens Per Property Per Hour | 40,000 | 400,000 |
Core Tokens Per Project Per Property Per Hour | 14,000 | 140,000 |
Core Concurrent Requests Per Property | 10 | 50 |
Core Server Errors Per Project Per Property Per Hour | 10 | 50 |
For additional limits, please reference analytics property quotas. |
As seen above, there are many benefits to leveraging the Google Analytics Data API (GA4), especially when it comes to ease of use and capabilities. For this reason, the Google Analytics Data API (GA4) is typically used to export historical data if BQ wasn’t/isn’t connected or for a majority of KPI reporting. However, it is important to note that if the data is going to be accessed heavily, historical data access beyond what the GA4 data retention setting allows is required, or accessing raw data is pivotal due to advanced analytic needs, then the BigQuery Export is probably the best option.
BigQuery Export (GA4)
BigQuery Export provides the most granular access to data being collected by any organization. With the move from Universal Analytics (UA) to GA4, one of the best product enhancements for standard licensed accounts was the ability to connect GA4 to BQ without the need of a Google Analytics 360 license. The best way to describe BQ probably comes from Google itself: “BQ is a serverless and cost-effective enterprise data warehouse that works across clouds and scales with your data. Use built-in ML/AI and BI for insights at scale.”
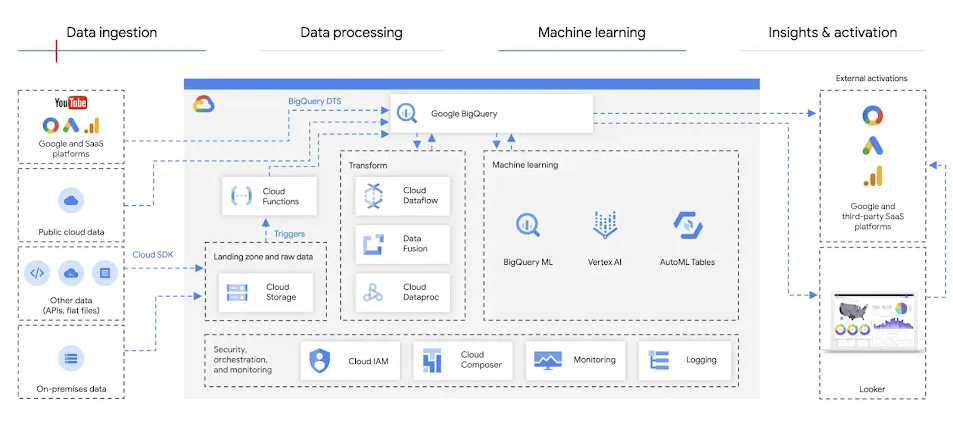
The projects that would be most ideal are large, advanced projects that will likely require data manipulation or a large historical time period. Other projects that might also benefit from BigQuery are standard licensed accounts that don’t have a need to buy a Google Analytics 360 license, but wish to be able to report on data for longer than 14 months (the longest data retention option for standard licensed accounts).
- Pros:
- Access to the raw data collected from the site.
- Is not limited to GA4 data retention settings.
- Can be leveraged to by-pass third-party servers via server-side tag management, thus making the data and process more privacy-centric and durable.
- Organizations/teams can utilize advanced analytic engineering capabilities to explore the data and perform advanced user analysis via the user ID or the customer ID.
- Provides an ability to create ML/AI models without providing access to a third-party system.
- Feed a real-time dashboard with up-to-date information to inform campaign needs.
- Enables an environment of offline + online data stitching in a flexible, durable manner (data blending is an option for a majority of other tools, but is typically more limited).
- Cons:
- Requires an understanding of Structured Query Language (SQL) to build/create queries.
- Has a cost associated with the storing and querying of data.
- Takes longer to stand up and build necessary queries for reporting and analytic needs.
- Are some limitations to what data can be seen in the GA4 UI and what is seen in BQ as the data in BQ requires some manipulations.
The benefits of BQ, even if you already use another data warehouse, are large as it provides one of the only places where organizations can access their backend user IDs sent to GA4 to help to tie together the full user journey. There is also an additional benefit that the organization owns all of their data that is collected with access to the full, raw data stream on a first-party server (parameter and user property names/values are still limited to the same character limits as seen in Google Analytics). This allows the organization to adhere more easily to privacy regulation and utilize advanced analytics capabilities.
Ability | File Download | GA Data API (GA4) | BigQuery Export (GA4) |
---|---|---|---|
Easy to access and utilize data | X | X | |
Immediate historical data access | X | X | |
No additional cost associated (from Google) | X | X | |
Does not require coding or mapping to match data available ing GA4 UI | X | X | |
Tool and programming language agnostic API | X | ||
Viable way to extract large amounts of GA4 data | X | X | |
Easy access to update and change data sets with little redo of the process | X | X | |
Raw data stream is accessible | X | ||
Not directly restricted by the GA4 UI settings (data retention, user_id, etc.) | X | ||
Unaffected by high cardinality and unregistered dimensions | X | ||
Data stored and accessed on first-party servers | X | ||
Flexible and durable solution to combine online and offline data | X | ||
Advanced analytics techniques can be leveraged | X |
Conclusion
In closing, it is important to understand what data is available and how to go about accessing the necessary data. In doing so, it is important to understand both the size, scope, and success factors of the project to ensure the proper tools are used in the first place. If you are currently trying to decide the best route to extract and leverage your GA4 data or you have identified what the best route is but would like to discuss it further, reach out! Here at InfoTrust, we are all about creating great partnerships, solving complex problems, and making miracles happen.